GTC 2025 Conference Summary
Wow, what an event! The NVIDIA GTC 2025 conference, held March 17-21 in San Jose, showcased the latest advancements in AI, accelerated computing, and their applications across a range of industries industries. This year’s event, described as the “Super Bowl of AI,” highlighted the remarkable progress in generative AI, agentic AI, and physical AI, with a particular focus on tokens as the foundation of inference. Jensen Huang, NVIDIA’s CEO, even said he’s going to have to grow San Jose to keep hosting an ever growing conference!. I was particularly interested in the idea of tokens now detached (in a way) from language or images, synthetic data generation, AI for medical and materials research, and robotics for everyday tasks.
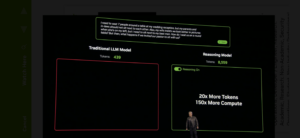
Tokens: The Building Blocks of AI
So let’s start with the new perspective on tokens as the fundamental building blocks of AI. In his keynote, Huang emphasized how tokens have “opened a new frontier” in AI development. Tokens transform raw data into meaningful insights, enabling AI systems to generate responses, analyze scientific data, and reason through complex problems. And before we get into a discussion about reasoning, no it’s not human reasoning, but it is reasoning nonetheless—stay tuned.
Huang addressed inference as the process of generating tokens and described it as “the ultimate extreme computing problem.” The ability to produce tokens efficiently is crucial for AI systems’ responsiveness and utility. No doubt this is important to the revenue equation, which Huang was quick to point out: “Inference is token generation by a factory, and a factory is revenue and profit generating,” But this is interesting from the perspective of taking evolution in our hands. In fact, this can be summarized by something Eric Steinberg, from Magic.dev, mentioned in the Beyond Prediction session: “I think reinforcement learning is good… It’s very hard to build superintelligence by training on data generated by humans.” (Yes, think on that for a bit.)
The computation requirements for token generation have increased dramatically with the advent of agentic AI and reasoning capabilities. Current models are generating 100 times more tokens than anticipated just a year ago. This explosion in token generation necessitates more powerful computing infrastructure to maintain AI system responsiveness.
For example, Huang demonstrated how a reasoning model generated over 8,600 tokens to solve a complex wedding seating problem, compared to around 400 tokens for a simple one-shot response from a traditional language model. This increase in tokens, combined with the need for faster computation, has driven the need for more sophisticated AI infrastructure.
To address these challenges, NVIDIA introduced NVIDIA Dynamo, described as “the operating system of an AI factory.” Dynamo manages the complex operations involved in token generation, including pipeline parallel, tensor parallel, expert parallel operations, and workload management. As an open-source tool, Dynamo aims to optimize token generation across different workloads and configurations.
Synthetic Data Generation and the Path to Superintelligence
A significant portion of the conference focused on how synthetic data generation is paving the road to more advanced AI systems. As Huang explained, training AI models faces two fundamental challenges: obtaining sufficient high-quality data and overcoming the limitations of human-in-the-loop training.
NVIDIA showcased several approaches to synthetic data generation that address these challenges:
Reinforcement Learning with Verified Results
One breakthrough highlighted at the conference is using reinforcement learning with verified results to train AI models. This approach leverages existing knowledge (like mathematical principles or puzzle solutions) to generate millions of examples for training. AI models are then rewarded as they improve at solving problems step by step.
“We have hundreds of these problem spaces where we can generate millions of different examples and give the AI hundreds of chances to solve it step by step,” Huang explained. This technique has enabled the generation of trillions of training tokens, vastly exceeding what would be possible with human-labeled data.
Digital Twins and Simulation for AV Development
NVIDIA demonstrated how Omniverse and Cosmos platforms are accelerating autonomous vehicle (AV) development through synthetic data generation. The process involves model distillation, closed-loop training, and 3D synthetic data generation.
In model distillation, knowledge transfers from a slower, more intelligent “teacher” model to a smaller, faster “student” model that can run efficiently in a vehicle. For closed-loop training, log data is transformed into 3D scenes for simulated driving scenarios, allowing the testing of trajectory generation capabilities without real-world risks.
The 3D synthetic data generation component enhances AV adaptability by creating detailed driving environments from log data, maps, and images. This approach provides diversity in training scenarios while closing the sim-to-real gap, as one presenter summarized: “Use AI to recreate AI.”
Scaling Laws and the Computing Requirements
The conference emphasized how synthetic data generation has changed our understanding of AI scaling laws. NVIDIA revealed that computation requirements for today’s agentic AI models are “easily 100 times more than we thought we needed this time last year.”
This increased demand is driven by two factors: AI models generating more tokens for reasoning (up to 100 times more) and the need for faster computation to maintain responsiveness. NVIDIA’s projections showed that Blackwell GPU shipments in their first year will significantly exceed the peak year of Hopper GPU shipments, reflecting the industry’s response to these demands.
AI for Medical and Materials Research
GTC 2025 showcased numerous applications of AI in advancing medical research and materials science, highlighting how computational methods are accelerating discoveries in these fields.
Digital Twins in Biomedicine
Professor Peter Coveney from University College London presented his work on computational biomedicine, particularly focusing on digital twins for personalized medicine. While digital twins are still nascent in biomedicine, they offer the potential to evaluate individuals based on their own data rather than population statistics.
“The issue is to get away from using AI in a population sense and claim that’s dealing with personalized medicine,” Coveney explained. “What it actually amounts to is building a large set of data on other people and using it to predict how you’re going to behave, which clearly isn’t personalized medicine.”
His team has developed HemeLB, a code that can model and simulate the entire human vasculature from head to toe. Running on supercomputers with up to 80,000 GPUs, this application enables unprecedented detail in cardiovascular simulation. By connecting organ models like the heart to the vasculature, researchers can study the coupling between blood flow and vital organs.
Coveney emphasized that GPU computing has transformed what’s possible in computational biomedicine by enabling high-resolution simulations that run fast enough for interactive use. Additionally, the integration of AI techniques with physics-based models helps accelerate simulations while maintaining scientific accuracy.
Human Brain Mapping at Cellular Resolution
Professor Mohanasankar Sivaprakasam from IIT Madras delivered an impressive talk on his team’s groundbreaking work imaging and mapping whole human brains at the cellular level. Over the past five years, his Brain Center at IIT Madras has undertaken the ambitious task of understanding the cellular and connectivity structure of the human brain, similar to work previously done by the Allen Brain Institute with mouse brains. Recently, they released the first set of detailed brain image volumes at full cellular resolution, representing the largest collection of open-source human brain data available to researchers worldwide.
“We are not only making this data fully public, we are absolutely willing to work with anybody to scale and accelerate this at various levels,” Sivaprakasam explained. The project has already acquired close to 300 meticulously preserved post-mortem human brains, generating petabytes of ground-truth data. To make this massive dataset accessible, the team has developed AI-powered tools that allow researchers to navigate through brain structures with unprecedented detail. Speaking at 3 AM from his time zone, Sivaprakasam highlighted that this work represents a significant advancement in neuroanatomy, which he noted had somewhat stagnated in recent decades with the advent of MRI technology. The cellular-level detail his team has captured goes far beyond conventional millimeter-resolution imaging, allowing researchers to explore the estimated hundreds of billions of cells in the human brain, including both neurons and support cells. This resource will be invaluable for understanding brain structure and function, with potential applications ranging from studying neurological disorders to developing more sophisticated AI models inspired by human neural architecture.
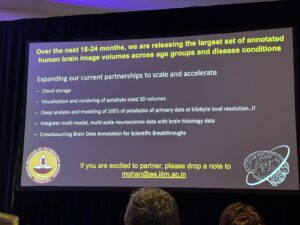
Accelerating Drug and Materials Discovery
The conference featured discussions on AI-accelerated materials discovery, with a focus on batteries and drug development. Chao-Chan Hu of SES AI demonstrated how his company is using AI to revolutionize battery development by mapping a “molecular universe” of 100 million potential molecules. Here’s an illustration of the molecular universe they are exploring.
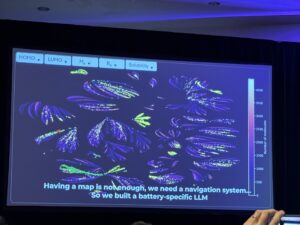
Using AI, SES AI reduced computational time for molecular property analysis from over 8,000 years (using CPUs) to just 2 months (using H100 GPUs and AI methods). This acceleration has allowed them to discover new molecules that significantly improve battery performance, enabling higher energy density batteries for electric vehicles and robots.
In drug discovery, presenters highlighted how NVIDIA’s BioNeMo framework is being used to accelerate research for diseases like amyotrophic lateral sclerosis (ALS). By leveraging generative AI techniques, researchers can reduce the drug discovery phase by up to 50%, creating new opportunities for treating challenging conditions.
Cell-free DNA and Cancer Diagnosis
Advances in cell-free DNA analysis for cancer detection were presented as a promising approach for early diagnosis. As tumors die, they shed DNA into the bloodstream, which can be isolated and analyzed using sequencing techniques to identify mutations.
This method allows for more regular monitoring of cancer dynamics, potentially detecting recurrence earlier than traditional methods. Speakers emphasized the importance of making this technology more accessible by developing efficient computational methods for processing the complex genomic data involved.
Robotics for Everyday Tasks
GTC 2025 dedicated significant attention to robotics, particularly how physical AI is enabling a new generation of robots that can assist in everyday tasks. Jensen Huang introduced the concept of “physical AI” as AI that understands the physical world, including concepts like friction, inertia, cause and effect, and object permanence.
NVIDIA Isaac Groot N1
A key announcement was NVIDIA’s Isaac Groot N1, a generalist foundation model for humanoid robots. Built on synthetic data generation and learning in simulation, Isaac Groot features a dual system architecture for both fast and slow thinking, allowing it to generalize across multiple embodiments and tasks.
The platform utilizes NVIDIA’s Omniverse and Cosmos technologies to create detailed simulations for robot training. By learning in virtual environments before deployment in the real world, robots can develop advanced capabilities while minimizing physical testing risks.
Social Robotics and Human-Robot Interaction
Professor Oya Asadikutan from King’s College London presented her work on social robotics and human-centered AI. Her research focuses on developing algorithms that enable robots to interact seamlessly with humans and their environment.
“Human behavior is rich, diverse, and often unpredictable,” Asadikutan noted. “To tackle these challenges, we must integrate multiple fields, not just robotics and AI, but also social sciences, such as behavioral psychology and ethics.”
One project from her lab focuses on teaching robots unspoken social rules, such as not interrupting conversations between humans. Her team developed an egocentric dataset to capture conversational groups from a robot’s perspective and created a graph neural network-based method for detecting these groups. This approach was integrated with reinforcement learning to enable robots to exhibit advanced social awareness.
Asadikutan emphasized the potential applications of such robots in supporting individuals with limited mobility and reducing anxiety in pediatric care settings. Her work demonstrates how multidisciplinary approaches combining AI, robotics, and social sciences can create more effective and acceptable robotic assistants.
Disney’s Robotic Character Platform
The conference included a session on Disney’s robotic character platform, which is redefining entertainment robotics from the ground up. Disney is building artist-centric tools that provide creative control of dynamic character motion, enabling the rapid design and deployment of animated robotic characters.
By combining advanced robotics with AI-driven motion planning, Disney aims to create more engaging and realistic character interactions for visitors. This application demonstrates how robotics is extending beyond industrial and healthcare settings into entertainment and storytelling.
Challenges in AI Data and Training
Throughout the conference, speakers addressed the fundamental challenges in AI development, particularly regarding data acquisition and training methodologies.
The Data Problem
As Jensen Huang highlighted, AI is a data-driven approach that requires digital experience to learn from. However, obtaining sufficient high-quality data remains a significant challenge. Speakers discussed various approaches to addressing this issue:
- Data curation and preprocessing: NVIDIA’s NeMo Curator for non-English languages was presented as a tool for creating high-quality text corpora for languages like Spanish and French, addressing the challenge of limited or imbalanced datasets.
- Synthetic data generation: Beyond autonomous vehicles, synthetic data generation was shown to benefit fields ranging from medical imaging to robotics, creating diverse training scenarios without real-world limitations.
- Privacy-preserving techniques: Federated learning for medical data was discussed as a way to leverage distributed datasets while maintaining privacy, an essential consideration for sensitive data.
Training Without Human in the Loop
The second major challenge identified was training models without heavy reliance on human feedback. Huang emphasized the need for AI to learn at “superhuman rates” that exceed what human supervision can provide.
Reinforcement learning emerged as a key approach to this challenge. By setting up environments where AI can learn through trial and error with automated feedback, models can generate their own training data through exploration. This approach has proven particularly effective in robotics and reasoning tasks.
Chain-of-thought reasoning was highlighted as a significant advance in AI capabilities. Models are now being trained to break down problems into steps, apply different approaches, and verify their own answers. This self-verification process improves reliability while reducing dependence on human evaluation.
AI Infrastructure and Computing Advancements
A substantial portion of the conference focused on the infrastructure required to support the next generation of AI models.
NVIDIA’s AI Infrastructure Roadmap
Jensen Huang unveiled NVIDIA’s roadmap for AI infrastructure, showcasing a systematic approach to scaling up computing capabilities before scaling out. Key announcements included:
- Blackwell architecture: Currently in full production, the Blackwell architecture represents a fundamental transition in computer design, with liquid cooling and disaggregated MVLink for improved performance.
- Blackwell Ultra: Coming in the second half of 2025, offering 1.5x more FLOPS, memory, and bandwidth compared to Blackwell.
- Vera Rubin: Planned for the second half of 2026, featuring NVLink 144, a new CPU with twice the performance of Grace, and new GPU, networking, and memory technologies.
- Rubin Ultra: Scheduled for the second half of 2027, with NVLink 576 enabling extreme scale-up capabilities with 15 exaflops of performance and 4.6 petabytes per second of bandwidth.
Energy Efficiency in AI Computing
Energy efficiency emerged as a critical factor in AI infrastructure development. Huang stated, “Energy is our most important commodity; everything is related ultimately to energy,” highlighting how data center revenues are fundamentally power-limited.
NVIDIA demonstrated that their Blackwell architecture is 25 times more efficient than Hopper in terms of tokens per second per megawatt, representing a significant advance in computational efficiency. The introduction of four-bit floating-point precision further improves energy efficiency by reducing the power needed for model operations.
The company also unveiled silicon photonic technology based on micro ring resonator modulators to dramatically reduce the power consumption of data center networking. This innovation could save tens of megawatts in large-scale AI deployments by eliminating traditional transceivers.
In Closing
GTC 2025 painted a picture of an AI industry at an inflection point, with foundational advances in tokens, synthetic data generation, medical and materials research applications, and robotics: these developments are creating a future where AI can reason, understand the physical world, and assist humans in increasingly sophisticated ways. I was skeptical about this until I learnt to see tokens as semantic units in AI–hence my coined phrase: “synthetic evolution.”
The integration of AI across industries is accelerating, from autonomous vehicles and healthcare to entertainment and enterprise computing. As Jensen Huang noted, “AI and machine learning have reinvented the entire computing stack,” fundamentally changing how we approach computation and problem-solving.
While significant challenges remain, particularly in energy efficiency and training methodologies, the technologies showcased at GTC 2025 demonstrate the industry’s commitment to addressing these obstacles. With continued advances in synthetic data generation, model architecture, and computing infrastructure, the path toward more capable AI systems seems to be taking us to a new frontier.
The conference’s emphasis on interdisciplinary collaboration—bringing together experts from computer science, medicine, materials science, robotics, and social sciences—highlights the importance of diverse perspectives in advancing AI capabilities. As these fields continue to converge, we can expect even more transformative applications that enhance human potential and address global challenges.